Note
Go to the end to download the full example code.
A single-frequency inversion (sEIT container)ΒΆ
Full processing of one frequency of one timestep of the data from Weigand and Kemna 2017 (Biogeosciences).
This example uses the sEIT container to load multi-frequency data from the original measurement data.
imports
import os
import numpy as np
import reda
import reda.utils.geometric_factors as geom_facs
from reda.utils.fix_sign_with_K import fix_sign_with_K
import reda.importers.eit_fzj as eit_fzj
define an output directory for all files
output_directory = 'output_single_freq_inversion_sEIT'
import the sEIT data set
seit = reda.sEIT()
seit.import_eit_fzj(
'data/bnk_raps_20130408_1715_03_einzel.mat',
'data/configs.dat'
)
Constructing four-point measurements
Summary:
a b ... frequency rpha
count 67375.000000 67375.000000 ... 67375.000000 67375.000000
mean 17.907532 20.831169 ... 2577.580109 473.723981
std 10.333167 10.677015 ... 8258.716055 2729.735973
min 1.000000 2.000000 ... 0.462963 -3141.592649
25% 10.000000 12.000000 ... 29.411765 -3131.399200
50% 17.000000 22.000000 ... 122.222220 5.510219
75% 27.000000 30.000000 ... 700.000000 3139.297772
max 37.000000 38.000000 ... 45000.000000 3141.592652
[8 rows x 7 columns]
with reda.CreateEnterDirectory(output_directory):
# export the data into CRTomo-style data files. Each frequency gets its own
# file
seit.export_to_crtomo_multi_frequency('result_raw_data')
# just for demonstration purposes, data could be imported from this
# directory:
# create a new sEIT container
seit_temp = reda.sEIT()
seit_temp.import_crtomo('result_raw_data')
# delete it to prevent any confusions
del(seit_temp)
Summary:
a b ... frequency rpha
count 67375.000000 67375.000000 ... 67375.000000 67375.000000
mean 17.907532 20.831169 ... 2577.580109 473.723981
std 10.333167 10.677015 ... 8258.716055 2729.735973
min 1.000000 2.000000 ... 0.462963 -3141.592649
25% 10.000000 12.000000 ... 29.411765 -3131.399201
50% 17.000000 22.000000 ... 122.222220 5.510219
75% 27.000000 30.000000 ... 700.000000 3139.297772
max 37.000000 38.000000 ... 45000.000000 3141.592652
[8 rows x 7 columns]
this is a container measurement, we need to compute geometric factors using numerical modeling Note that this only work if CRMod is available
settings = {
'rho': 100,
'elem': 'data/elem.dat',
'elec': 'data/elec.dat',
'sink_node': '6467',
'2D': True,
}
k = geom_facs.compute_K_numerical(seit.data, settings)
seit.data = geom_facs.apply_K(seit.data, k)
fix_sign_with_K(seit.data)
SETTINGS
{'rho': 100, 'elem': 'data/elem.dat', 'elec': 'data/elec.dat', 'sink_node': '6467', '2D': True}
2D modeling
apply correction factors, as described in Weigand and Kemna, 2017 BG
corr_facs_nor = np.loadtxt('data/corr_fac_avg_nor.dat')
corr_facs_rec = np.loadtxt('data/corr_fac_avg_rec.dat')
corr_facs = np.vstack((corr_facs_nor, corr_facs_rec))
seit.data, cfacs = eit_fzj.apply_correction_factors(seit.data, corr_facs)
apply some filters import IPython IPython.embed()
seit.filter('r < 0')
seit.filter('rho_a < 15 or rho_a > 35')
seit.filter('rpha < - 40 or rpha > 3')
seit.filter('rphadiff < -5 or rphadiff > 5')
seit.filter('k > 400')
seit.filter('rho_a < 0')
seit.filter('a == 12 or b == 12 or m == 12 or n == 12')
seit.filter('a == 13 or b == 13 or m == 13 or n == 13')
# seit.filter_incomplete_spectra(flimit=300, percAccept=85)
seit.print_data_journal()
seit.print_log()
--- Data Journal Start ---
2024-12-09 10:30:04.799523
A filter was applied with query "r < 0". In total 543 records were removed
A filter was applied with query "rho_a < 15 or rho_a > 35". In total 13919 records were removed
A filter was applied with query "rpha < - 40 or rpha > 3". In total 10016 records were removed
A filter was applied with query "rphadiff < -5 or rphadiff > 5". In total 14451 records were removed
A filter was applied with query "k > 400". In total 3619 records were removed
A filter was applied with query "rho_a < 0". In total 0 records were removed
A filter was applied with query "a == 12 or b == 12 or m == 12 or n == 12". In total 1003 records were removed
A filter was applied with query "a == 13 or b == 13 or m == 13 or n == 13". In total 934 records were removed
--- Data Journal End ---
2024-12-09 10:30:04,755 - reda.main.logger - INFO - Data sized changed from 67375 to 66832
2024-12-09 10:30:04,763 - reda.main.logger - INFO - Data sized changed from 66832 to 52913
2024-12-09 10:30:04,770 - reda.main.logger - INFO - Data sized changed from 52913 to 42897
2024-12-09 10:30:04,777 - reda.main.logger - INFO - Data sized changed from 42897 to 28446
2024-12-09 10:30:04,782 - reda.main.logger - INFO - Data sized changed from 28446 to 24827
2024-12-09 10:30:04,787 - reda.main.logger - INFO - Data sized changed from 24827 to 24827
2024-12-09 10:30:04,793 - reda.main.logger - INFO - Data sized changed from 24827 to 23824
2024-12-09 10:30:04,799 - reda.main.logger - INFO - Data sized changed from 23824 to 22890
export normal data to volt.dat file note that this is not required for the subsequent code and could be completely removed !!!
with reda.CreateEnterDirectory(output_directory):
seit.export_to_crtomo_one_frequency('volt.dat', 70.0, 'nor')
alternatively: directly create a tdman object that represents a single-frequency inversion with CRTomo
import crtomo
grid = crtomo.crt_grid('data/elem.dat', 'data/elec.dat')
tdman = seit.export_to_crtomo_td_manager(grid, frequency=70.0, norrec='nor')
# set inversion settings
tdman.crtomo_cfg['robust_inv'] = 'F'
tdman.crtomo_cfg['mag_abs'] = 0.012
tdman.crtomo_cfg['mag_rel'] = 0.5
tdman.crtomo_cfg['hom_bg'] = 'T'
tdman.crtomo_cfg['d2_5'] = 0
tdman.crtomo_cfg['fic_sink'] = 'T'
tdman.crtomo_cfg['fic_sink_node'] = 6467
# run the inversion, use the given output directory to store the CRTomo
# directory structure for later use
# only invert if the output directory does not exists
outdir = '{}/tomodir_inversion'.format(output_directory)
if not os.path.isdir(outdir):
tdman.invert(
catch_output=False,
output_directory=outdir,
)
else:
tdman.read_inversion_results(outdir)
print('Statistics of last iteration:')
print(tdman.inv_stats.iloc[-1])
This grid was sorted using CutMcK. The nodes were resorted!
Rectangular grid found
Attempting inversion in directory: output_single_freq_inversion_sEIT/tomodir_inversion
Using binary: /usr/bin/CRTomo_dev
Calling CRTomo
Inversion attempt finished
Attempting to import the results
Reading inversion results
is robust False
Info: res_m.diag not found: output_single_freq_inversion_sEIT/tomodir_inversion/inv/res_m.diag
/home/runner/work/crtomo_tools/crtomo_tools/examples/02_simple_inversion
Info: ata.diag not found: output_single_freq_inversion_sEIT/tomodir_inversion/inv/ata.diag
/home/runner/work/crtomo_tools/crtomo_tools/examples/02_simple_inversion
Statistics of last iteration:
iteration 3
main_iteration 3
it_type DC/IP
type main
dataRMS 0.9981
magRMS 0.9
phaRMS 9.0
lambda 561.3
roughness 0.3254
cgsteps 70.0
nrdata 354.0
steplength 9.0
stepsize 0.000066
l1ratio NaN
Name: 14, dtype: object
evolution of the inversion
fig = tdman.plot_inversion_evolution()
with reda.CreateEnterDirectory(output_directory):
fig.savefig('inversion_evolution.png')
# the statistics are stored in a data frame
print(tdman.inv_stats)
print(tdman.inv_stats.columns)
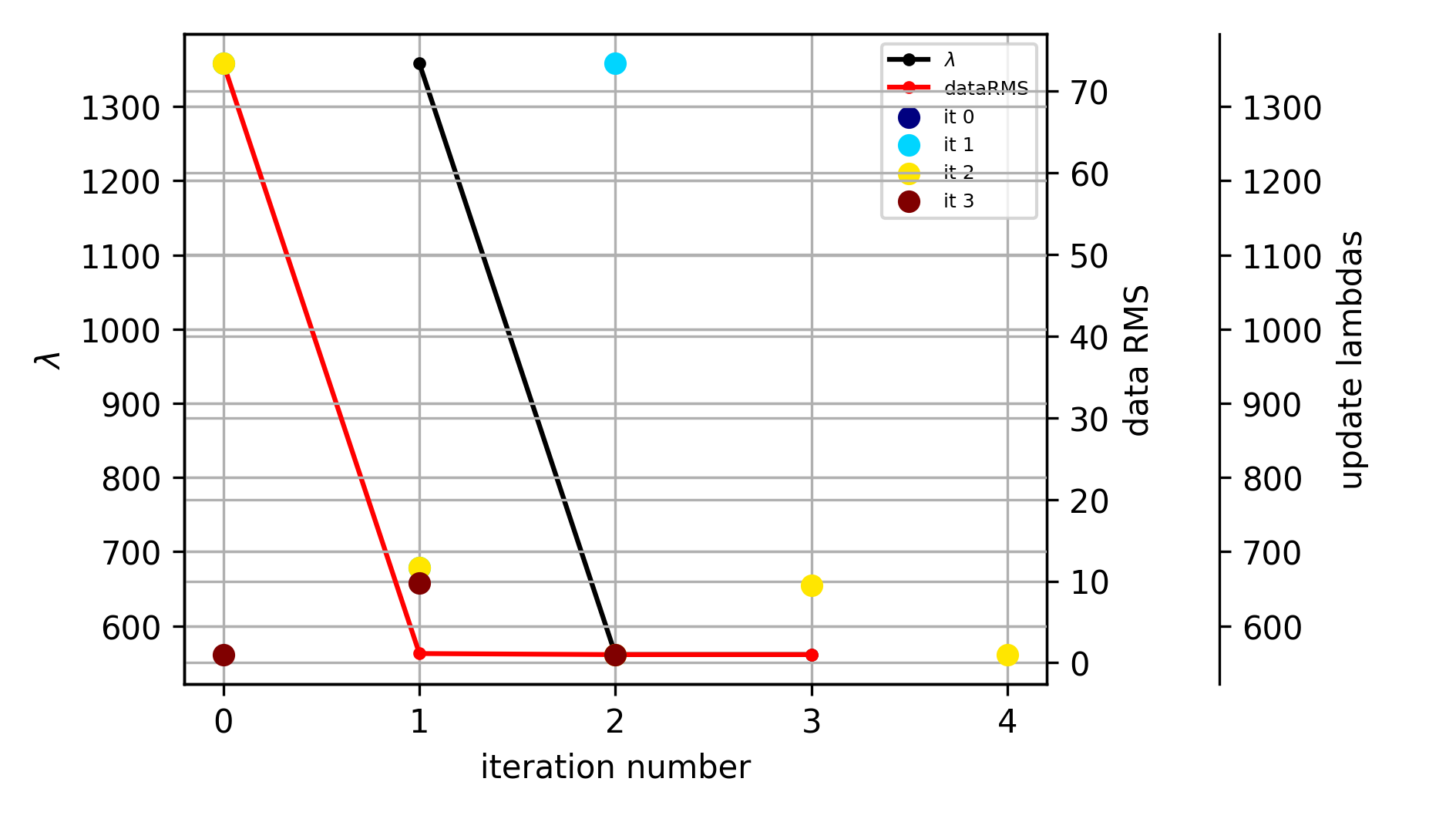
#############################
0
Empty DataFrame
Columns: [iteration, main_iteration, it_type, type, dataRMS, magRMS, phaRMS, lambda, roughness, cgsteps, nrdata, steplength, stepsize, l1ratio]
Index: []
#############################
1
iteration main_iteration it_type ... steplength stepsize l1ratio
1 1 1 DC/IP ... 1.0 7420.0 NaN
2 2 1 DC/IP ... 1.0 7340.0 NaN
3 3 1 DC/IP ... 0.5 3710.0 NaN
[3 rows x 14 columns]
#############################
2
iteration main_iteration it_type ... steplength stepsize l1ratio
5 0 2 DC/IP ... 1.0 11.00 NaN
6 1 2 DC/IP ... 1.0 9.52 NaN
7 2 2 DC/IP ... 1.0 9.19 NaN
8 3 2 DC/IP ... 1.0 9.46 NaN
9 4 2 DC/IP ... 0.5 4.59 NaN
[5 rows x 14 columns]
#############################
3
iteration main_iteration it_type ... steplength stepsize l1ratio
11 0 3 DC/IP ... 1.0 0.0656 NaN
12 1 3 DC/IP ... 1.0 0.1060 NaN
13 2 3 DC/IP ... 0.5 0.0328 NaN
[3 rows x 14 columns]
iteration main_iteration it_type ... steplength stepsize l1ratio
0 0 0 DC/IP ... NaN NaN NaN
1 1 1 DC/IP ... 1.0 7420.000000 NaN
2 2 1 DC/IP ... 1.0 7340.000000 NaN
3 3 1 DC/IP ... 0.5 3710.000000 NaN
4 1 1 DC/IP ... 8.0 7421.000000 NaN
5 0 2 DC/IP ... 1.0 11.000000 NaN
6 1 2 DC/IP ... 1.0 9.520000 NaN
7 2 2 DC/IP ... 1.0 9.190000 NaN
8 3 2 DC/IP ... 1.0 9.460000 NaN
9 4 2 DC/IP ... 0.5 4.590000 NaN
10 2 2 DC/IP ... 9.0 8.314000 NaN
11 0 3 DC/IP ... 1.0 0.065600 NaN
12 1 3 DC/IP ... 1.0 0.106000 NaN
13 2 3 DC/IP ... 0.5 0.032800 NaN
14 3 3 DC/IP ... 9.0 0.000066 NaN
[15 rows x 14 columns]
Index(['iteration', 'main_iteration', 'it_type', 'type', 'dataRMS', 'magRMS',
'phaRMS', 'lambda', 'roughness', 'cgsteps', 'nrdata', 'steplength',
'stepsize', 'l1ratio'],
dtype='object')
evolution of data misfits
fig = tdman.plot_eps_data()
fig = tdman.plot_eps_data_hist()
# eps data is found here:
tdman.eps_data
r = tdman.plot.plot_elements_to_ax(
tdman.a['inversion']['rmag'][-1],
plot_colorbar=True,
cmap_name='jet_r',
)
with reda.CreateEnterDirectory(output_directory):
r[0].savefig('rmag.png', bbox_inches='tight')
r = tdman.plot.plot_elements_to_ax(
tdman.a['inversion']['rpha'][-1],
plot_colorbar=True,
cmap_name='jet_r',
)
with reda.CreateEnterDirectory(output_directory):
r[0].savefig('rpha_last_it.png', bbox_inches='tight')
Total running time of the script: (3 minutes 14.362 seconds)